Microsoft recently unveiled Visual ChatGPT, a novel model that merges visual foundation models (VFMs) like Transformers, ControlNet, and Stable Diffusion with ChatGPT. Moreover, the system allows for ChatGPT interaction that goes beyond language.
What is Visual ChatGPT?
Visual ChatGPT is a system that combines various VFMs such as Transformers, ControlNet, and Stable Diffusion with ChatGPT. This new model allows users to interact beyond language, transmitting and receiving images, complex visual inquiries, and visual editing instructions requiring the collaboration of multiple AI models.
Previously, ChatGPT has been limited to linguistic training and has been unable to process or produce images. While adept at specialized tasks, visual foundation models have been limited in their versatility. Visual ChatGPT solves this problem by combining these models to create a multimodal conversational model that can perceive and generate visual information.
The new system is built on top of ChatGPT, making use of a Prompt Manager that connects ChatGPT and these VFMs. The Prompt Manager manages the histories, priorities, and conflicts between several visual foundation models and changes different kinds of visual information into language format to help ChatGPT understand.
Related News:
Opera Browser to Integrate ChatGPT-Powered AI Content Services for Enhanced Browsing Experience
Top 10 industries that can use ChatGPT
ChatGPT rival from Google is called Bard: Check details
How does Visual ChatGPT work?
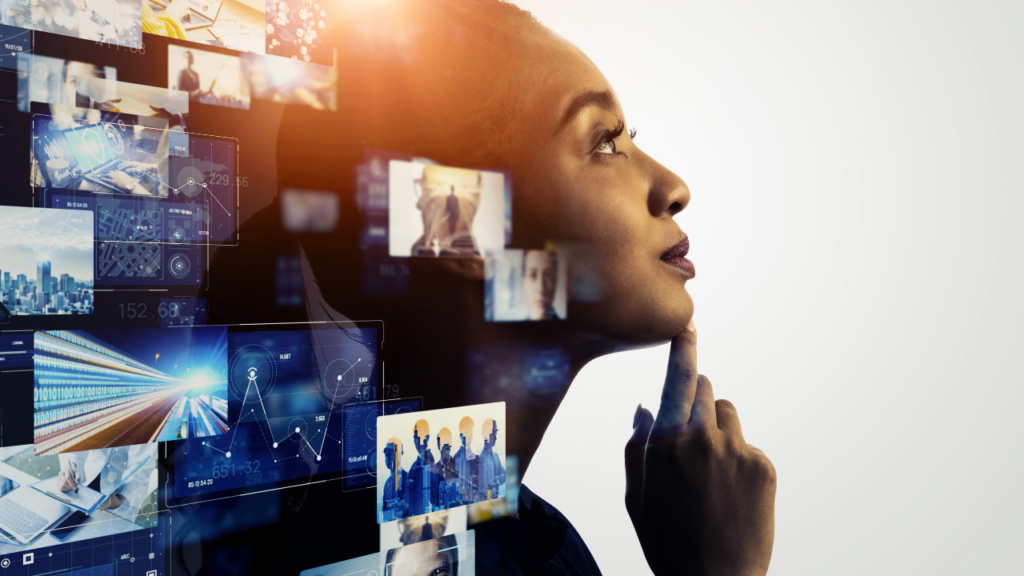
Visual ChatGPT is an advanced machine learning model that combines natural language processing (NLP) and computer vision techniques to enable machines to understand and generate text in response to visual input. It is an extension of the popular ChatGPT model trained on massive amounts of data and can generate human-like responses to text-based prompts.
The addition of computer vision capabilities to ChatGPT enables Visual ChatGPT to analyze visual input such as images, videos, and other forms of visual data and generate contextually relevant responses. It does this by using a combination of convolutional neural networks (CNNs) to extract visual features from the input data and transformers to process the extracted features and generate responses.
For example, a user uploads a picture of a dancing lady on the stage with a set of instructions like “draw an illustration of a lady dancing.”
With the help of the Prompt Manager, Visual ChatGPT starts the execution of linked visual foundation models. In particular, it uses a depth estimation model to figure out the depth information, a depth-to-image model to turn the depth information into a picture of a dancing lady, and a style transfer VFM based on a stable diffusion model to make the image look like an illustration.
Potential Applications of Visual ChatGPT
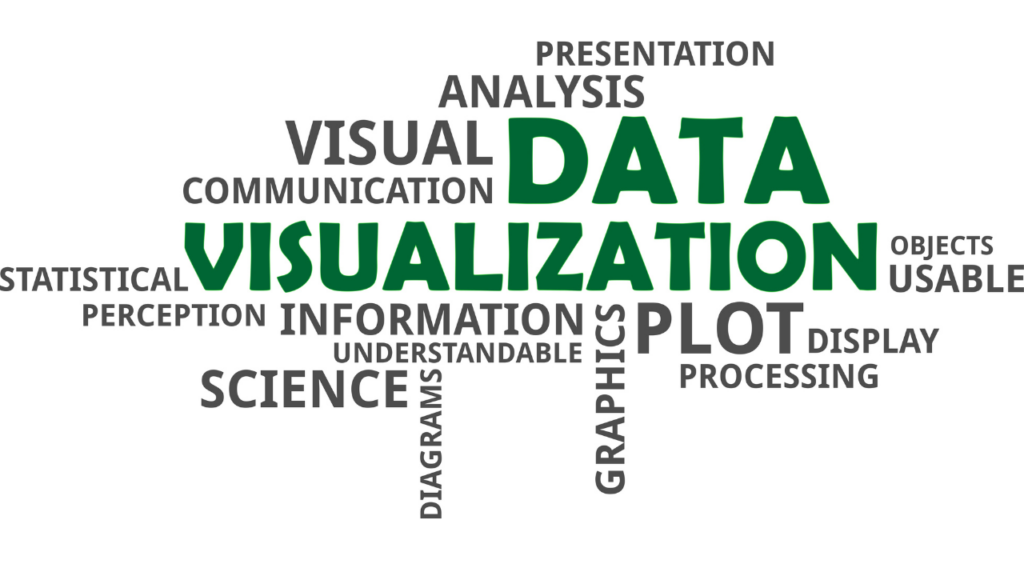
Visual ChatGPT has a wide range of practical applications across different domains. Here are some examples:
- Customer Service: Visual ChatGPT can be used as a customer service chatbot that understands customer text and image input. It can quickly and accurately respond to customer queries, complaints, and feedback.
- E-commerce: Visual ChatGPT can be integrated into e-commerce websites to assist customers with product recommendations, sizing, and styling advice based on the customer’s input and image inputs of their preferences.
- Education: Visual ChatGPT can be used in educational applications to provide personalized learning experiences. It can help students with questions and problems about coursework by providing explanations, additional resources, or suggesting videos and tutorials.
- Healthcare: Visual ChatGPT can be used in healthcare to assist patients with symptom diagnosis and triage. The chatbot can provide preliminary diagnoses and advice or refer them to a specialist by analysing patient images and text inputs.
- Entertainment: Visual ChatGPT can be used in entertainment applications such as gaming or social media. It can generate responses that incorporate both text and image information to provide a more immersive and engaging experience.
Developing Visual ChatGPT has not been without its challenges, including combining two very different types of models, obtaining and labelling enough training data, and integrating the outputs from both modalities effectively. It represents a significant advance in the development of chatbots that can understand and respond to multimodal inputs. It can revolutionise how we interact with machines and provide a more natural and intuitive interface for users.